Challenges and Solutions in Scaling Data Annotation for AI
- Barb Ferrigno
- Dec 13, 2023
- 6 min read
Updated: Jan 4, 2024
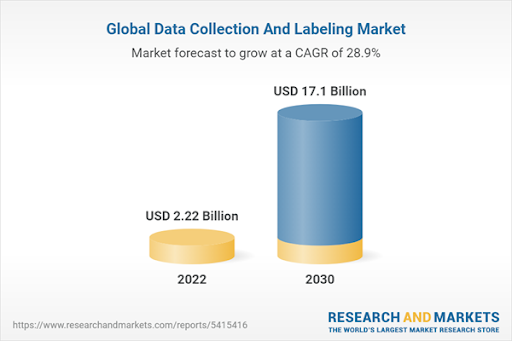
AI is creating valuable growth opportunities for all industries, be it eCommerce, gaming, or finance. However, to push the capabilities of AI/ML models or to make them more advanced, you need to train them on more representative training datasets, raising the need for consistent data collection and labeling.
As AI applications expand across industries, the demand for high-quality annotated data is skyrocketing. A recent report by ResearchandMarkets forecasts that the global data collection and labeling market is projected to reach a valuation of $17.10 billion by 2030.
This surge in demand, however, brings with it a series of challenges that hinder the efficient scaling of data annotation operations. Let's take a closer look at these challenges and how businesses can overcome them to train their AI models more efficiently.
Key challenges involved in scaling data annotation for AI/ML models with possible solutions
Managing large-scale data annotation is not a simple task for organizations. Several internal and external obstacles can make the process ineffective and time-consuming. These challenges include:
Collecting large amounts of data for labeling at a speed
The more complex the AI model, the more data it needs to be trained on. Organizations need to collect this data quickly and consistently to avoid delays in creating new NLP models or refining the existing ones. However, this is where most organizations face challenges. Limited resources, a lack of specialized skills, and the struggle to find credible data sources can make it difficult for companies to collect relevant data for annotation at a large scale.
Possible solutions:
To address these challenges, businesses can:
Thoroughly examine their internal data repositories to identify potential sources of data that can be labeled for AI models’ training
Partner with reputable data providers or crowdsourcing platforms to get access to diverse and high-quality datasets. However, before utilizing data provided by such entities for labeling, it is crucial to assess their data collection practices and the quality of datasets.
Leverage synthetic data generation techniques to create large, diverse datasets for annotation without relying on real-world data collection
Maintaining quality and consistency of training data
Errors in data labeling can lead to inaccurate predictions or outcomes, costing businesses both money and reputation. Getting high-quality data for creating training datasets isn’t sufficient alone, accurate labeling of that data is equally important to maintain the effectiveness of AI models. However, in large-scale data annotation processes, maintaining consistency in data quality and accuracy can be significantly challenging for organizations. As the volume of data to be trained increases, so does the complexity of the project, making it more prone to errors.
Possible solutions:
To maintain the integrity of their training datasets, businesses can:
Define clear guidelines for data quality standards. These guidelines must include acceptable error rates, data formats, and labeling conventions to be followed by data annotators.
Establish a multi-level QA process to identify inaccurate, inconsistent, duplicate, and missing data to fix at the initial stages.
Develop a feedback loop post-labeling to continuously monitor and evaluate the quality of annotated data.
Mitigating human bias during data labeling
Human bias is common to occur when working on large amounts of training datasets. Biases can be introduced by human data annotators due to various reasons, such as:
Lack of knowledge or subjective understanding of certain concepts, languages, or accents
Choice of sampling data that doesn't represent the real-world distribution of the target population
Ambiguous or poorly defined data guidelines leading to subjective or inconsistent labeling
When AI models get trained on biased datasets, they perpetuate these biases in their predictions, affecting their overall efficiency.
Possible solutions:
To minimize the risk of introducing human biases in training datasets, businesses can:
Hire data annotators from diverse backgrounds or subject matter experts to ensure a comprehensive understanding of the data
Define clear guidelines for data labeling to reduce subjective interpretations and inconsistencies
Leverage manual review, statistical analysis, and automated bias detection tools to identify potential biases in the data
Ask multiple data annotators to label the same dataset and compare their results
Complying with data security guidelines
Strict adherence to data security regulations can be significantly challenging for businesses during large-scale data annotation. This is especially true when the data they are using is sensitive or includes personally identifiable information (PII) like names, addresses, or social security numbers. Not protecting such information can lead to data breaches, legal penalties, and reputational & financial loss for organizations.
There can be several reasons due to which organizations can put data security at risk, such as:
A sheer volume of training data that can be difficult to securely store and manage
Data sharing with third-party service providers for labeling, raising concerns about data leaks or unauthorized access
Lack of awareness about specific data security regulations that apply to their industry
Not considering data security as their top priority or lacking the necessary actions required to comply with these guidelines
Possible solutions:
To comply with data security regulations, organizations can:
Develop an effective data governance framework outlining how the data will be collected, shared, stored, and managed to prevent unauthorized access
Train their staff on applicable data security policies and processes including how to identify and report suspicious activity
Conduct regular data security audits to identify and address potential vulnerabilities
Encrypt sensitive data at rest and in transit to prevent data breaches or misuse. Additionally, businesses can implement multi-factor authentication and role-based access control, to restrict access to authorized personnel.
Evaluate data handling practices of the service provider when outsourcing data annotation services, to ensure that data is protected throughout the labeling process. It is better to partner with a reputable or ISO-certified data annotation company that follows strict data security guidelines to protect your sensitive data.
Handling a vast workforce and maintaining efficiency
As data annotation needs increase when a project scales, so does the team responsible for labeling training datasets. Managing such vast teams of data annotators while maintaining both accuracy and efficiency can be a significant challenge for organizations. Finding the right people for your large-scale data annotation project or providing them with the necessary training can be time-, resource-, and money-intensive. Additionally, maintaining effective communication across the team can be another big hurdle without the right collaboration tools or frameworks in place.
Possible solutions:
To ensure seamless collaboration and efficiency between data annotation team members, organizations can:
Leverage project management tools like SmartSheet, Wrike, and Asama to build custom workflows, manage project schedules, collaborate with the team in real-time, and monitor and track team members’ productivity
Organize team meetings and knowledge-sharing sessions to promote collaboration and continuous improvement
Invest in cloud-based annotation platforms that can handle large volumes of data and support a growing workforce
Define performance metrics for data annotators that include number of annotations they have completed per day and the quality score for each task
Getting access to advanced data labeling tools
Data annotation involves various tasks, from collecting data to labeling it. For each step, you need both cutting-edge tools and well-trained professionals. Data can come in various formats, such as text, images, audio, and video. Each format has its unique challenges and requires specialized tools and techniques for annotation. A tool that works well for labeling images may not be suitable for video or text annotation.
This diversity of data formats and the need for specialized annotation tools can make the entire process challenging for organizations. The high cost of labeling tools and the need for ongoing workforce training can make organizations hesitant to invest in advanced infrastructure.
Possible solutions:
To overcome these challenges, organizations can:
Invest in versatile data labeling tools that can support various data types and formats effectively
Adopt open-source data annotation tools, such as LabelBox, LabelMe, and Dataloop, that are more affordable and easy to use than proprietary software
Outsource data support services for AI/ML models to a reputable third-party provider who is equipped with large teams of skilled professionals and advanced infrastructure to seamlessly handle your growing requirements. They can help you stay productive and cost-efficient while handling all the intricacies of your large-scale data annotation project.
Bonus tip: When outsourcing data labeling services to a company, always check their past work, client reviews, hiring models, price plans, and data security measures for optimal results.
Summing up
Scaling data annotation for AI models presents challenges in terms of maintaining quality, managing costs, ensuring data security, and addressing industry complexities, but solutions exist to overcome these hurdles. Businesses can seamlessly scale their data annotation projects for enhanced growth by leveraging best practices such as outsourcing data labeling services, implementing robust security measures, and utilizing cutting-edge tools. As the demand for data collection and labeling is projected to soar in the coming years, organizations must embrace a hybrid approach that integrates automated data labeling tools with human oversight to thrive in this dynamic landscape.
Comments